By Bruce Davey, William Rulla & Allison Nau, Lexis Nexis
Most insurance distributors now use price optimisation as a key part of their pricing toolkit. However, nearly all current price optimisation models are based on the assumption that a customer receives a single offer from the distributor and makes a single yes/no decisions based on that offer. This is increasingly not the case, especially on aggregator sites and on more sophisticated direct websites/call centre processes. What we must explore as an industry is the motivation for competing offer models and three key considerations.
1. Making multiple offers significantly increases business performance over a single offer.
Multiple offers come in many flavours. Some distributors have multiple insurance brands with which to badge their product. Some offer different products (e.g. “Light” or “Premium” coverage products in addition to a “Standard” product). Some offer flexible configuration – e.g. quoting prices for several excess levels, and with or without various inclusions and add-ons.
These strategies provide substantial advantage. Ask a multi-brand insurer if they would shut down all but one brand, or a multi-product insurer if they should shut down all but one product, and in most cases you’ll get an overwhelming ”No!.” Different consumers have different brand preferences, different product preferences and needs, so making more than one option available makes it more likely that you have something they want. We don’t want to confuse the consumer with a ridiculous array of offers, but in general keeping the set of offers that get reasonable conversion rates is materially better than a single offer.
2. Single offer optimisations draw the wrong conclusions when multiple offers strongly compete.
Although a standard optimisation analysis can be conducted in isolation on individual offers, such an analysis makes the assumption that anyone rejecting your brand because you raised your price is going to a competitor, or more insidiously, any new volume you’ve obtained by lowering price is coming from outside your company.
Unfortunately, it may be your own brand and products which are absorbing the brunt of the action, rendering the individual offer analysis a waste of effort at best, and potentially harmful to your overall business.
Using single offer techniques causes companies to set prices too low for any of its offers in segments where the offers strongly compete as it mistakes the other offers for the competition. This needlessly gives away significant profits.
3. Competing offer modelling & optimisation software uses a sophisticated set of novel techniques to correctly model the interaction between offers.
Choice modelling is quite different from modelling individual metrics like loss or even elasticity. It is rarely covered in undergraduate programmes and requires different model forms than would be typically found in risk modelling software. The object being modelled is now a vector of probabilities, and customer switching behaviour is governed by a matrix of cross elasticities, which in turn are functions of the array of prices by offer. A cross elasticity is the change in volume of one offer as a function of the change in price on another.
Unfortunately, the most obvious and perhaps natural candidate for choice modelling, the multinomial logit, allows no flexibility whatsoever in cross elasticities - it requires them to be fixed by conversion rates. In aggregator’s channel business, where conversion rates are tiny, this effectively assumes no offers strongly compete. This is exactly the situation that regular binomial models work fine. In practice however, many offers do strongly compete. An insurer tends to use the same loss models for all their offers, so their offers will tend to be ranked closely together. Similarly a broker tends to have the same panel members for many of their offers, causing theirs to be clustered.
The notion – that some offers compete more strongly with each other – is known as preference inhomogeneity. A more complex model form is needed to do any reasonable job of taking it into account.
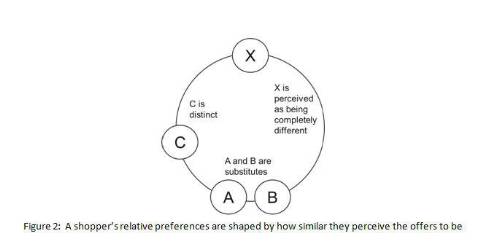
On the other hand, arbitrarily ‘flexible’ analytic forms don’t guarantee natural a priori assumptions about a shopper’s behaviour, e.g. that cross elasticities are directionally correct. A model form appropriate for price optimisation should embody this and other well-studied econometric principles: essentially a good model should align with the notion that a shopper is trying to maximise some underlying notion of economic utility.
Finally, training flexible choice models which do adhere to econometric principles is quite difficult compared to more standard e.g. GLM risk models because of the general complexity of the problem, and the prevalence of local optima, so special computational considerations are required.
However more general choice models can be built which have all the nice properties outlined above, and none of the bad. Capturing your portfolio’s preference information accurately, adhering to econometric principles, and training quickly enough to support optimisations and ‘what-if’ analyses allows the competing offer solutions to accurately model the give-and-take between your brands – so you can maximise your overall business objective without pitting your own brands against each other.
|