By Wan Hsien Heah, Partner and Consultant at Barnett Waddingham
Our process with human intelligenceOver time, the graduates will learn from those refinements and hopefully develop their own reserving philosophy. There is no written rulebook, just a learning process built by trial and error as well as intuition over several years.
Our process with AI
In this case, we tested a neural network model as it is one of the closest ways to simulate how a brain works. The process of training a neural network model is much faster. We trained it on factors including premiums, claims developments, and Solvency II class. We used data going back to 2012 and, for Lloyd’s data, going back even further to 1996.
All in, we fed hundreds of triangles and tens of thousands of data points into the model - certainly more triangles than we would ever put a graduate through. We did this in a matter of hours using thousands of modelling iterations and tens of variations of model structure itself, an example of which is shown in the diagram below (the number and size of layers for those familiar with neural networks).
For those of you who do not speak “neural network” – we basically trained it A LOT.
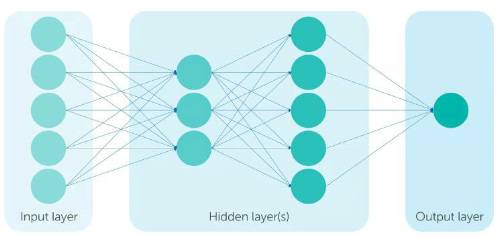
Who achieved the best results overall?
With a typical graduate, we hope they achieve an accuracy of at least 50% i.e. their work has some semblance to the final answer at least half the time.
When we compared the outcome of the neural network model, we found that the accuracy (defined by the linear correlation coefficient between the predicted and actual results) was around 40%. Accuracy varied by line of business with the more stable lines being more predictable as one would expect. It is close, but no cigar.
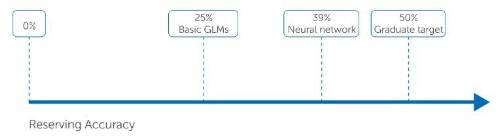
Why is this the case?
So, why was the neural network model not better than the graduate despite all the training we gave it?
Well, the first obvious answer is that we did not run the model on enough variables. This is partly true. With natural intelligence, we learn to examine, observe and think about more than just the data. For example, we consider soft factors such as market conditions, bias, economic context, verbal updates and the fundamentals of how a line of business would behave. These are things which may, in theory, be easy to integrate within a neural network but would be complicated to achieve and risks identifying trends that are purely coincidental but not real (we sometimes call this Simpson’s paradox).
Further, it would not be too outlandish to suggest that bias plays a role in shaping our perception of how we reserve. With neural networks, the bias is built into the training data. With natural intelligence, we sometimes lean on gut instinct i.e. bias outside of the data. This is not something one simply teaches a model.
In addition, when a graduate starts with the previous year’s model, they are at least re-using judgements and assumptions made in the previous year. For the more volatile lines, it is possible that those judgements could survive a year. The neural network model always starts fresh with no supervision or course correction along the way. Our data set also included more volatile lines of business.
What does this mean?
Firstly, it means that it is highly unlikely for human actuaries to be entirely replaced by AI any time soon, particularly where there are complex, soft factors at play with the reserves.
Secondly, neural networks are probably overkill for reserving. Simple Generalised Linear Models (GLMs) were usually outperformed by the neural network. However, chain ladder models, even simpler than GLMs, tended to achieve better results without the complexity (brushing aside that chain ladder models are pseudo GLMs). Sometimes, simpler is better.
"But most importantly, it shows that the training of future actuaries, both formal and on the job, is pivotal to ensuring that we achieve the best results."
The importance of spending time on soft factors, context and external trends cannot be understated. This is the reason why actuarial trainees across the market continue to grow and develop into successful actuaries, despite there not being a ‘rulebook’ on how to navigate reserving.
Ed Hundleby and Dan Ritchie also contributed to this article.
|